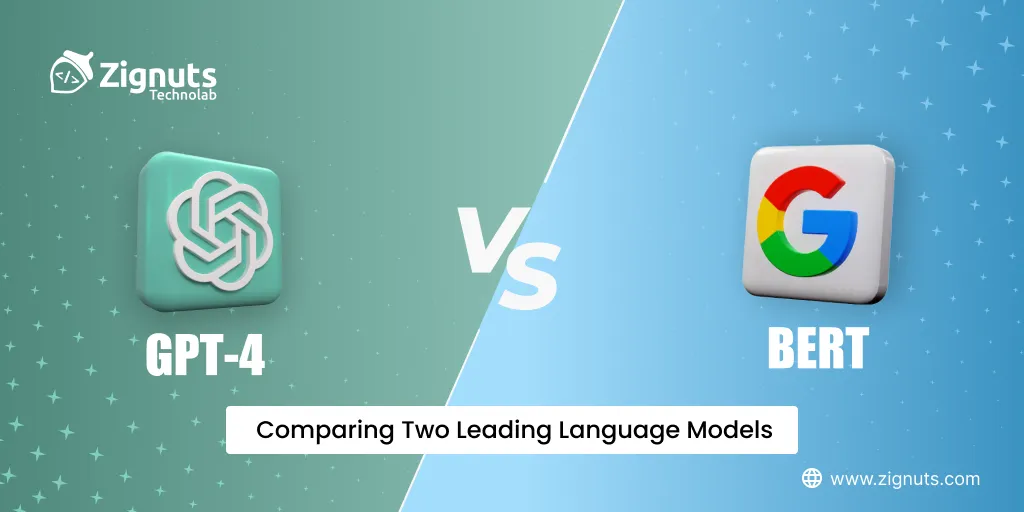
In the ever-evolving field of artificial intelligence and natural language processing (NLP), two models stand out for their groundbreaking achievements: GPT-4 (Generative Pre-trained Transformer 4) and BERT (Bidirectional Encoder Representations from Transformers). Both have set new benchmarks in NLP tasks, yet they serve distinct purposes and excel in different areas. This article delves into a detailed comparison of GPT-4 and BERT, complete with real-life applications and personal insights.
GPT-4 is the latest iteration in OpenAI's series of generative pre-trained transformers. Its primary capability lies in generating coherent, contextually accurate text. By using a decoder architecture, GPT-4 can predict the next word in a sentence based on the words that precede it, making it extraordinarily efficient for text generation tasks such as writing essays, creating dialogues, and even coding.
GPT-4 usually requires fine-tuning for specific applications but performs exceptionally well even without extensive domain-specific training. On the other hand, BERT often necessitates fine-tuning to excel in specialized tasks, even though it is incredibly strong out of the box in various comprehension-based tasks.
When it comes to benchmarks like the GLUE (General Language Understanding Evaluation), BERT shines in tasks like sentence classification and question answering. Conversely, GPT-4 excels in more creative endeavors where generating coherent, human-like text is the goal.
GPT-4 has revolutionized the content creation landscape. For instance, platforms like Jasper and Copy.ai utilize GPT-4 to help marketers draft compelling copy, blog posts, and social media updates within minutes. From personal experience, I’ve used an AI-writing tool powered by GPT-4 to draft complex technical articles. The output was impressively accurate and required minimal editing, saving me countless hours.
Companies are employing GPT-4-powered chatbots to handle customer support queries, reserving human agents for more complicated issues. These chatbots can generate natural-sounding responses, providing a smooth customer experience. A notable example is OpenAI's integration into Zendesk, enabling automated support that closely mimics human interaction.
BERT, developed by Google, is fundamentally different. It leverages a bidirectional encoder, which means it reads entire sequences of text at once rather than from left to right or right to left. This enables BERT to grasp the full context of a word within a sentence, making it incredibly effective for comprehension-based tasks such as question answering and sentiment analysis.
BERT has dramatically improved the way search engines like Google understand user queries. Instead of focusing on individual keywords, BERT grasps the entire context of the query, leading to more accurate search results. In personal observation, Google searches have become significantly more intuitive and contextually relevant, providing answers to complex queries that conventional keyword-based searches struggled with.
BERT models have been implemented in healthcare for tasks such as predicting patient outcomes and understanding medical inquiries. Fine-tuned versions of BERT have been able to parse clinical notes and research papers, assisting healthcare professionals in making better-informed decisions. For instance, projects like Google Health are leveraging BERT to summarize patient records, making it easier for doctors to review a patient's medical history.
Having interacted extensively with both models in various applications, I find each brings unique strengths to the table. For creative and generative tasks, GPT-4 is unmatched. Its ability to produce coherent, contextually relevant text is nothing short of spectacular. I've used GPT-4 for generating content ideas, writing detailed articles, and even composing poetry. The experience has been highly fulfilling, showcasing how far AI has come in mimicking human creativity.
On the flip side, BERT is a maestro of comprehension-based tasks. I've worked on projects that required fine-tuning BERT for specific purposes like sentiment analysis and domain-specific question-answering. The level of understanding BERT exhibits makes it a powerful tool in interpreting and responding to contextual nuances. This has been invaluable in customer feedback analysis and sentiment tracking, helping businesses understand their customers better.
Despite its prowess, GPT-4 isn't without flaws. One of the primary issues is the "hallucination problem." The model can sometimes generate text that, while coherent, is factually incorrect. This necessitates careful review and editing, especially in applications requiring high accuracy.
BERT imposes computational challenges. Its bidirectional nature requires more memory and processing power, making it less feasible for real-time applications. Additionally, fine-tuning BERT for specific tasks can be resource-intensive and requires significant preparatory work.
As we look towards the future, the potential applications for both GPT-4 and BERT are immense. The former is well-positioned to continue making strides in creative fields like content generation, entertainment, and even virtual companionship. Companies like OpenAI are consistently working on minimizing biases and improving factual accuracy, which will only enhance GPT-4’s utility.
Conversely, BERT and its descendants (like RoBERTa and DistilBERT) will likely dominate tasks requiring deep understanding and context. Innovations focusing on reducing computational overhead while maintaining the model's integrity will make BERT more accessible for various applications, including real-time services.
Choosing between GPT-4 and BERT boils down to the specific needs of a project. If your aim is generating human-like text, GPT-4 should be your go-to. However, if the task involves understanding and interpreting text, BERT is the superior model.
From personal experience and observations, I can attest to the versatility and robustness of both models. Leveraging their strengths in appropriate contexts can lead to significant advancements in various domains, from healthcare and education to customer service and content creation.
In summary, both GPT-4 and BERT exemplify the marvels of modern NLP, each excelling in unique ways to bring us closer to a future where AI not only serves but also understands and creates alongside humans. The journey is far from over, and as technology continues to evolve, so will the capabilities of these extraordinary language models.
Looking to implement AI-driven solutions for your business? Hire ChatGPT developers to build cutting-edge applications tailored to your needs.
Portfolio
Recent
Projects
Explore Projects